Ron Beck, Marketing Strategy Director, Aspen Technology, talks about how hybrid models combine AI, first principles and domain expertise to deliver a comprehensive, accurate model of new and complex processes quickly.
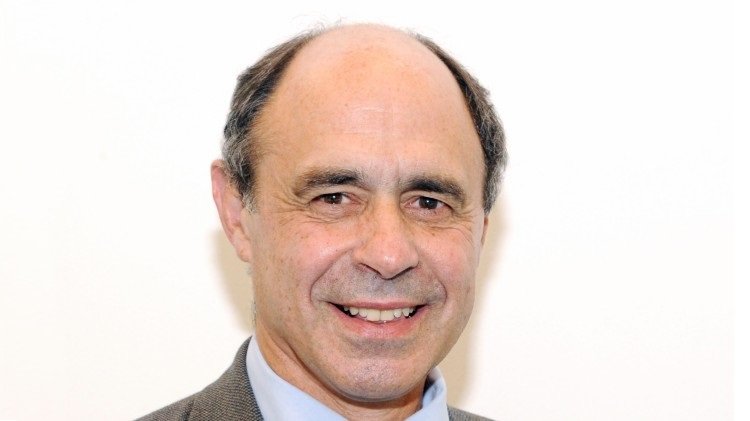
AI and machine learning are rapidly emerging as tools that can greatly accelerate the ability to employ plant data both to calibrate first principles models and to create data-based models of phenomena and processes quickly. Especially in the Middle East, where the UAE has been one of the first countries to draft a National Artificial Intelligence Strategy 2031, and PwC expects the potential impact of AI in the Middle East to reach US$320 billion. Hybrid models combine AI, first principles and domain expertise to deliver a comprehensive, accurate model of new and complex processes quickly.
Dr Karuna Potdar, Vice President, Reliance Industries, said, “Aspen Hybrid Models are a major advance in the field of chemical engineering. Hybrid Models are a major step forward in bringing together AspenTech’s process models and machine learning, and are a game changer in process engineering and plant improvement.”
With hybrid models, users can model processes and assets that cannot easily be modeled with first principles alone. They provide a better representation of the plant, which keeps the model more relevant over a longer period of time. This reduces the barrier to entry for using modeling for asset optimization by requiring less effort and expertise. With the models in place, the connected worker becomes free to perform higher value-added and strategic work.
Today, we are seeing hybrid modelling capabilities increasingly deployed across software solutions through a model alliance approach, which synchronises fit-for purpose models in different functional areas needed to safely, reliably, sustainably and profitably operate an asset. An example of the model alliance is the use of reduced order unit models in planning, dynamic optimisation and online equipment monitoring, all derived from the same root refining unit operating data set and simulation model, achieving closed-loop production optimisation.
A Vision of Hybrid Modelling in the Process Industry
Democratising access to powerful and accurate models, across all sized assets and companies, is a crucial step in understanding how a specific process will behave or respond to unexpected change. As plants and their systems have increased in complexity, these models have become essential to operations.
Hybrid models combine AI and first principles to deliver a comprehensive, accurate model more quickly without requiring significant expertise. Machine learning is used to create the model leveraging simulation or plant data, while using domain knowledge including first principles and engineering constraints to build an enriched model – without requiring the user to have deep process expertise or become an AI expert.
Today, we are seeing the development of three types of hybrid models, which are all industry firsts, namely:
Type 1: AI-driven Hybrid Models
This approach uses machine learning to create an empirical model based on plant or experimental data, augmented with first principles (e.g. thermodynamic properties etc.), constraints (e.g. mass balance) and domain knowledge. A less-experienced user can rapidly generate a completely new predictive, more accurate model, fully democratizing AI. Users can now model processes and assets that cannot easily be modeled with first principles alone. Examples include complex reaction unit operations, new material processes and new technology processes.
Type 2: Reduced Order Hybrid Models
In this approach, machine learning is used to create an empirical model based on data from numerous simulation runs, augmented with constraints and domain expertise, to build a fit-for-purpose, high fidelity, performant model that is accurate within the range for which it has been trained, fully democratizing AI. With reduced order models, users can easily extend the scale of modeling from units to the entire site and synchronize the model across design, operations and maintenance. Examples include building value chain-wide models from crude oil input to finished chemical output, building rapidly deployable and compact models online at the edge, and embedding high accuracy models in the planning LP.
Type 3: First Principles-Driven Hybrid Models
This approach augments an existing first principles model with AI, using data from operations to calculate unknown variables and relationships not captured by the original model. Machine learning determines the unknown value and its relationships to continuously calibrate the model as conditions change. This approach is a natural extension to existing first principles models in many brownfield deployments globally; it is quick and easy to adopt and significantly increases accuracy. Examples include embedding AI-created but first principles-governed models for unique batch process units.
Here are some of the unique benefits hybrid models can provide.
1. Expanding Modelling’s Scope and Impact: Complex units often pose yield, performance and quality issues. Hybrid models enable process engineers to model several equipment types that are difficult or impossible to describe using first principles, such as specialty chemical reactor models. Hybrid models expand the scope of modeling from process to total sites, using reduced order hybrid models to make solving these large problems possible.
2. Democratising Modelling: Organizations have a high proportion of newer engineers today who all need to work off the same information. Hybrid models empower a typical process engineer to develop models for equipment and assets without expert modelling skills, using operational data and the built-in data science to develop reliable and fit for purpose models.
3. Creating Accurate, Fit for Purpose Models: Traditionally, different fit for purpose models have been used in different functional areas, making closing the loop a challenge. Now, reduced order hybrid unit models that represent complex behaviors simply but accurately, in the paradigm appropriate for planning, dynamic optimization and online equipment monitoring, can all easily be derived from the same root refining unit operating data set and simulation model, achieving closed-loop production optimization.
4. Better Sustaining Modelling’s Benefits: Since hybrid models have both data-driven and first principles components, they are closely tied to plant data and able to keep in synch with the asset operations closely as the operations evolve and therefore are able to sustain the benefits of modelling more than pure first principles modeling.
5. Accelerating Collaboration Between Disciplines: Reduced order modelling enables model alliance across disciplines. For example, planning models updated by hybrid models from rigorous reactor models in refining improve information sharing and collaboration.
We also foresee that hybrid, AI-powered models could fundamentally change how humans work, interacting with intelligent systems in the business. In place of spending many hours building and rebuilding models and handling data streams in largely manual spreadsheets workers will perform higher-level functions, making forward-thinking, data-driven decisions about what to do – thereby increasing overall accuracy, efficiency and performance while reducing system-level risk.
Looking ahead
AI is rapidly proving its ability to provide predictive insights in industrial domains. For AI to provide effective insights into process industry processes and equipment, domain expertise remains the key. Against this backdrop the emergence of hybrid modeling, which covers a wide range of high-business-value use cases, that create measurable value for operators and engineers in today’s environment, has a key role to play.
Today, process industry organisations are facing unprecedented challenges. To map a path forward for businesses amid demand uncertainty, feedstock and crude price volatility, sustainability pressures and global competition requires agility, insight and enterprise-wide analysis. To execute this in the midst of a wave of retirements, which subtract decades of experience, is even more challenging.
Now is the time for organisations to embrace digitalisation and AI in a way that provides a smooth path forward, building on the powerful tools they have used for decades, enabling more automation, actionable insights from the models, and remote use of these tools. This will democratise AI in the design, rapid evolution, operation of process industry units and plants.